Cerebras New Trillion Transistor Chip will Turbo-Charge ‘Brain-Scale’ AI
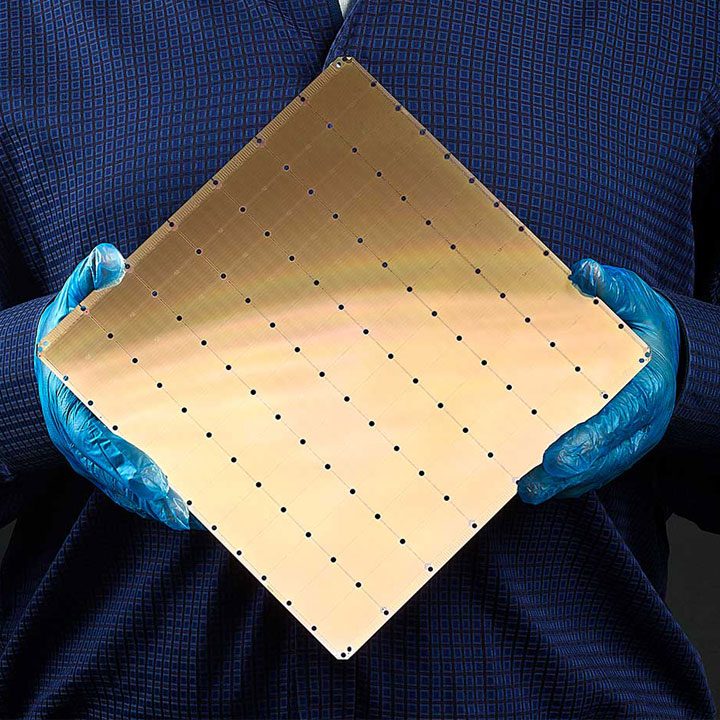
In 2019 Cerebras, a new chip startup company revealed a 1.2 trillion transistor chip the size of a dinner plate. The chip named Wafer Scale Engine was the worlds largest computer chip back then. In 2021, the company revealed the Wafer Scale Engine 2, a 2.6 trillion transistor chip that promises to turbo-charge ‘brain-scale’ artificial intelligence.
These enormously powerful chips will supercharge the development of artificial intelligence by enabling the training of neural networks with up to 120 trillion parameters. Currently OpenAI’s GPT-3 language model can handle 175 billion parameters and Google’s largest neural network to date contains 1.6 trillion parameters.
Cerebras is able to achieve this staggering level of chip capacity by approaching chip development differently from the traditional approach. Rather than splitting silicon wafers to make smaller chips, it simply makes one large chip. By creating one massive chip instead of individual chips, it makes the chip ideal for tasks that require large numbers of operations to be done in parallel.
One major drawback for large neural networks is moving around all the data involved in calculations. Most chips only have a limited amount of memory on-chip and it creates a bottleneck every time data is moved in and out. This problem limits the size of networks. The enormous 40gb capacity of the WSE-2 on-chip memory allows it to handle a staggering amount of information transfer on the largest of networks. In addition, the company has built a 2.4 Petabyte high-performance memory known as MemoryX which will supplement and function as on-chip memory.
Cerebras new approach keeps the bulk of the neural network data on the MemoryX unit with data streamed in during training. Prior to Cerebras new approach, the training data would be fed into the system with the bulk of the neural network stored on the chip. This new approach can train networks two orders of magnitude larger than anything that exists today.
With AI models increasing in size every year, it will likely be years before anyone can push the WSE-2 to its limits. Increasing parameter counts will undoubtedly lead to massive increases in performance and will bring us closer to answering the “holy-grail” question in AI circles of whether we can achieve general artificial intelligence by simply building larger neural networks or whether it requires further innovation.